Qualitative data analyses using longitudinal data
In-Brief:
The longitudinal studies are a type of survey that mainly uses the method of observation, which entails that they do not involve interfering with the subjects in any means.The purpose of this article is to provide a concise, broad and readily accessible overview of longitudinal Data analysis methods, aimed to be a practical guide for clinical investigation.
Traditional Qualitative data analyses methods
(1) Normal regression of the dependent variable on a time measure
(2) Qualitative data analysis services help in analysing a linear summary subject level number that indexes changes for each subject
(3) Sample qualitative data analysis plan helps in a general linear model approach with a known subject effect, should be reserved for quick, simple or preliminary analyses.
Advantages of Qualitative Data Analysis using longitudinal data
1. They are influential in determining variable patterns over time:
Because these studies require the use and collection of data in expand periods, they can determine patterns directly. Data analysis plan for qualitative research assist by utilizing them, it would be possible for researchers to know more about causation and effectiveness relationships and make connections in a more precise manner. Apart from this, remember that more data over more extended periods will allow for concise and better results. These studies are considered valuable for determining long-term changes and are unique in themselves when it comes to being able to provide useful data about these individual changes.
2. They can ensure focus and validity:
With a clear target, longitudinal studies would let researchers observe how a particular set of conditions or an end state would come to be. Statistical data analysis in qualitative market research helps to address this problem byutilizing actual recording that ensures a high level of validity.
3. They are instrumental in researching developmental trends:
They are used to study life probability throughout lifetimes or generations. It is so because, unlike cross-sectional studies where different individuals with similar characteristicscompared, longitudinal studies would track the same people, which means that the differences observed in a group will be less likely to be the result of a change or difference in culture across generations.
4. They are more potent than cross-sectional studies:
As they use the examination method without manipulating the state of the world, longitudinal studies have argued about having low power in terms of detecting causal relationships contrast with experiments. However, they are known to have higher power than cross-sectional studies when it comes to evading time-invariants and unobserved individual differences and when it comes to observing a particular event’s order, as they use same observations at individual levels.
5. They are highly flexible:
Quantitative data analysis in business research helps inlongitudinal studies that regularly observe to allow flexibility to occur. It means that the focus they use can shift while researchers are collecting data for the outcome.
6. They can provide high certainty when observing changes:
With their quality of being the ideal method to research developmental trends, these studies can monitor changes more preciseby making them as the standard option in various fields. In life science, for example, longitudinal studies are used to discover indicators of certain diseases, while in advertising, they are used to decide changes that a target has made in the behaviour of consumers who belong to its target audience and have seen the advertisement.
Disadvantages of Qualitative Data Analysis using longitudinal data:
Missing Data
Missing data is the most challenging issue as there is no universally accepted correction, and inappropriate ones can lead to negative consequences.Coding in qualitative research analysis assistance helps to overcome the missing data by filling incomplete data.
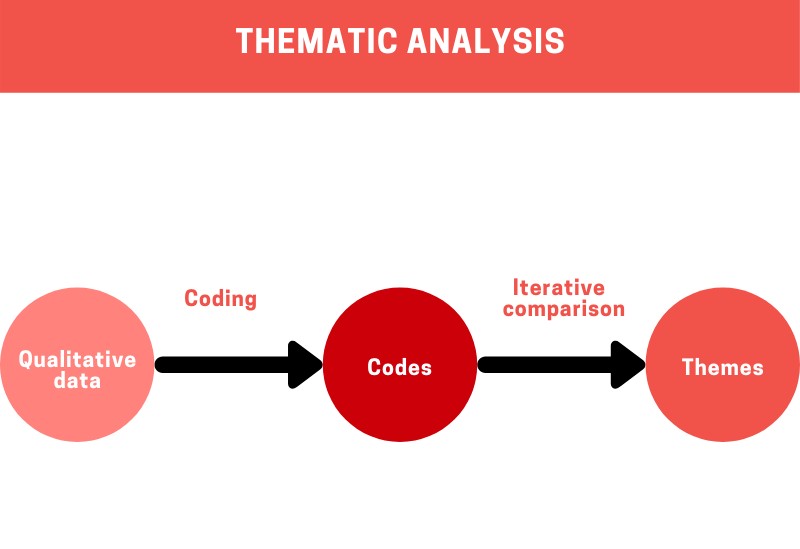
Impact of Missing Data
Missing data can decrease the study’s statistical power and increase bias. Statistical power improves whenever the study’s sample size increases or variability of the study’s outcome measure should be accurate. Unfortunately, missing data negatively impacts the sample size and variability. Qualitative research sample size calculator analyses that exclude participants with missing values inadvertently reduce the study’s sample size, potentially reducing the statistical power. Second, when participants who would have had extreme data values drop out, e.g., participants with very high or very low TMS, the variability of the study’s outcome measure is incorrectly underestimated.
Irregularly Timed Data
Qualitative Data Analysis Help for dissertation helps in longitudinal studies generally encourage regularly occurring visits for Data collection. But study participation frequency, and total study visits vary due to scheduling limitations and dropout. In our TMS example, individuals in the highormediumdisease category may have limited mobility because they have more severe disease as the study progresses and may miss scheduled visits.Qualitative research sampling design helpsthe analysis of longitudinal data by accounting for possible between-subject heterogeneity and within-subject correlation.
References:
- Tan, X., Shiyko, M. P., Li, R., Li, Y., &Dierker, L. (2012). A time-varying effect model for intensive longitudinal data. Psychological methods, 17(1), 61.
- Zeger, S. L., & Liang, K. Y. (1992). An overview of methods for the analysis of longitudinal data. Statistics in medicine, 11(14‐15), 1825-1839.
- Gibbons, R. D., Hedeker, D., &DuToit, S. (2010). Advances in the analysis of longitudinal data. Annual review of clinical psychology, 6, 79-107.
- Liang, K. Y., &Zeger, S. L. (1986). Longitudinal data analysis using generalized linear models. Biometrika, 73(1), 13-22.