How CPG Companies Can Leverage Predictive analyses to remain ahead of the competition
Introduction
The Consumer Packaged Goods (CPG) industry generates a massive amount of data these days. This information aids business executives in better understanding the competition and making choices that maximize brand awareness and profits. Furthermore, companies have access to large data sets and information from social networks, all of which can be categorized as big data. For CPG companies to obtain actionable insights, benefit from retail, and supply chain processes, they must spend appropriately, organize data, and analyse it. Big data and advanced analytics can predict changes in the CPG sector and establish a progressive approach to spearhead sustainable growth in a competitive global market. According to McKinsey, CPG companies that excel at revenue-growth management and data analytics are more likely to win and remain ahead of the competition.
In addition, Businesses who want to make profitable decisions are increasingly relying on data. Retailers examine the data because they have discovered that it has a positive impact on their businesses. The following are the cases in which data science contributes more to solving business problems and optimizing the different processes.
- Market-based analysis
- Warranty analysis
- Optimization of price
- Prediction of consumer behaviour
- Inventory management
- Building new product
- Sentiment analysis
- Predictive analytics for building marketing strategy
- Outlier or Fraud detection and so on.
Data Science in E-commerce
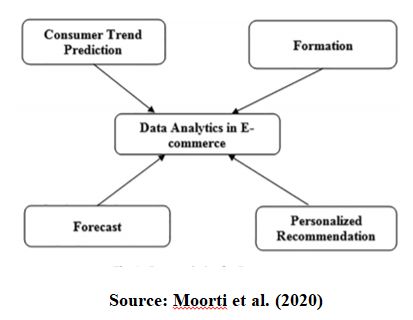
Source: Moorti et al. (2020)
In e-commerce, data analytics is critical. Nowadays, almost all e-commerce firms adopt analytic data techniques. It also aids in better stock management, the development of a strong supply chain, the analysis of data to identify fraud, the prediction of what lies ahead for you, the personalization of recommendations for your customers, the forecasting of inventory for the next season, the measurement
Various algorithms used by the e-commerce firms
- Various e-commerce firms used data analytics to compete. Data analytics techniques are applied in the e-commerce industry and their impact by comparing the sales in various seasons of an e-commerce seller. Suppose you are interested in understanding the influence of sales of the products in marketing through online reviews like amazon, flipkart, etc.
In such a case, the neural network technique will be useful to model the data. The neural network identifies the pattern and trend in the dataset with the hidden layers. The input node is the receiver that receives data files, as the name implies. The final information produced is stored in the output layer. Between the input and output layers are the secret layers. The hidden layers will receive feedback from the input layer’s neurons, and the interneuron relation strengths will store information. Then with the help of a supervised learning algorithm, it will analyse the data.[7]
- Modern service-focused architecture called Big Data Analytics Service-Oriented Architecture to increase business performance through big data analytics. big data analytics service provider requester, big data analytics service provider, and big data analytics service broker are the three major players. Sample data collected containing the demographic information of IT managers recruited from the national firm adopted the Structural Equation Modeling (SEM) to carry out the analysis. SEM consists of measurement and structural model. It combines confirmatory factor analysis (measurement model) and multiple regression analysis (structural model) between the constructs. SEM provides greater accuracy, and it is highly used in the marketing field. [2]
- Social media analytics explain the performance of the market and its impact on big data. In addition, with the collected employee’s data, it is identified that big data analytics positively affect performance in the market.[1]
- The defect inspection of textured surfaces in the manufacturing industry using the deep learning concept. For detecting the surface, a convolution neural network is used to defect in the surface area and identified that this data analytic method reduces the time and cost. [3]
- Optimizing algorithm to imitate the swarm behaviour of tunicates and tested the robustness of the proposed algorithm on six constrained engineering designs.
- Hybrid algorithm for solving economic dispatch problems. And found that this hybrid algorithm is highly efficient than other algorithms in the literature.
Types of Data
Data in CPG is broadly classified into two types they are
- Observational data – One can analyse the observational data to find the product’s performance and find a direction to introduce an Innovative new product in the market.
- Sales data – This data is used to identify the trends in the market sales, and it helps to decide the market price. It can be combined with promotional (activity) data to improve the business in a better manner.
Advantages of Analytics
Big data analytics has been adopted by many CPG businesses to enhance sales, frame business strategy, understanding customer behaviour, etc. The following tables show few advantages of big data analytics in various fields of CPG business.
Area | Benefits of data analytics |
Supply Chain Management | It gives improved efficiency and minimizes the expenses. |
Inventory Management | It helps to plan, forecast and optimize the inventory |
Marketing | It helps to identify the customer preferences about various brands of products. |
Consumer effectiveness | It helps to understand the customers buying behaviour and increase sales by analysing the brand value. |
Demographic decisions | It helps the researcher or marketer to develop strategies and helps to make complex business decisions. |
Conclusion and Future Scope
Investing in CPG data analytics generates exponential growth and earnings. Businesses must heavily invest in big data and advanced analytics. The accurate overview of ongoing operations in multiple retail locations is provided by monitoring CPG data. Measuring and monitoring this data as a long-term plan will help CPG companies make better resource allocation decisions and ensure that supply chain processes and solutions achieve the best possible results.
Future scope of predictive analytics can be adopted by CPG companies which include: finding the difference in the functionality of organizations, studying the factors which impact the market value with high dimensional data, customization of price for customers, integrating and merging the big data in CPG industry, etc.
References
- JQ. Dong, C.-H. Yang, Business value of big data analytics: A systems-theoretic approach and empirical test, Inform. Manage. 57 (1) (2020) 103 – 124, https:// doi.org/10.1016/j.im.2018.11.001.
- M. Ghasemaghaei, The role of positive and negative valence factors on the impact of bigness of data on big data analytics usage, Int. J. Inform. Manage. 50 (2020) 395–404.
- M. Garg, G. Dhiman, Deep convolution neural network approach for defect inspection of textured surfaces, J. Inst. Electron. Comput. 2 (1) (2020) 28–38.
- S. Kaur, L.K. Awasthi, A.L. Sangal, G. Dhiman, Tunicate Swarm Algorithm: A new bio-inspired based metaheuristic paradigm for global optimization, Eng. Appl. Artific. Intell. 90 (2020) 103541.
- G. Dhiman, MOSHEPO: a hybrid multi-objective approach to solve economic load dispatch and micro grid problems, Appl. Intell. 50 (1) (2020) 119–137.
- G. Dhiman, M. Soni, H.M. Pandey, A. Slowik, H. Kaur, A novel hybrid hypervolume indicator and reference vector adaptation strategies based evolutionary algorithm for many-objective optimization, Eng. Comput. (2020) 1–19.
- K. Moorthi, Gaurav Dhiman, P. Arulprakash, C. Suresh, K. Srihari. (2021). A survey on impact of data analytics techniques in E-commerce, Materials Today: Proceedings. Article in Press.