A detailed description in research planning/designing bioinformatics experiments
In general, a research design is an outline for planning the research and getting inference for the research questions. Every research design makes the decision about the type of data needed and collecting the same, time of research, the observations or respondents, hypotheses, and Data analysis. A research design determines the important parameters for the study and defines the criteria to evaluate the results. The key features of every research design are a good research methodology, the sample data, procedure of data collection and a proper statistical methodology to draw conclusions (Honaas, Altman, & Krzywinski, 2016).
Nowadays, statisticians are the main backbone of any type research especially they are involved in many ways of bioinformatics research. Statisticians and any statistical data analysis services in the experimental design are more important than any other research type such as simply doing any data analysis after the ata collection. In these bioinformatics research, statisticians or any other professional data analysis service reduces the errors and inefficiencies that reflect in the subsequent analyses and thus gives valid discoveries. However, in many situations, the statisticians faces a problem of understanding in bioinformatics, as they are not evolved in the biological area. This result in getting a help from the data analyst is quite slow in bioinformatics research and because of this mistake there are many false positive results arises in the experimental designs. Due to the computational challenges and inconvenience, various statistical analysis are not modelling the complete dataset and are often ad hoc procedures leaving uncertainty and increases the false conclusions in the data analysis. In 1930’s, R. A. Fisher, a famous statistician said that “To call in the statistician after the experiment is done may be no more than asking him to perform a post-mortem examination: he may be able to say what the experiment died of.” Thus, it is important to involve the statistical community as higher source to improve the efficiency of the research. The main objective of any experimental design is to obtain reproducible findings with accuracy with minimal resources. In this blog, we walk through the research design or plan in the field of bioinformatics experiments (Kerr & Churchill, 2001).
The classical view of every experimental design include determining the
- Objective of the study.
- Experiment conditions (treatments).
- measurement platform
- Biological sampling design.
- Biological and technical replicates.
- sequencing depth
- measurement design
- sequence of the study (inference can be generalizable or not)
- Carrying out the study
Let’s look at some of them in more detail.
1. Determining the Objective
Replication level should match the objective of the study. In the case of differential analysis, we usually compare the difference of mean between the treatments and replicates. Thus, one should decide the appropriate level of replication for the study. For instance, consider a study on tumor development in mice, and the scientist might decide to check for one tumor in every mouse. However, suppose mice can develop several types of tumors, then testing the sample mouse for two tumors and identify the variability (Altman & Hua, 2006).
Once we recorded the readings, next step is to conduct the statistical analysis. Suppose if we want to test the means of the same, then a t-test will be helpful. If the samples are heterogeneous, then a cluster analysis might serve the purpose. Thus, balancing the biological and statistical replicates is the main key in every designed experiment. In some cases, we may know the measurement error and in that case we don’t have to concentrate more on technical aspects. However, in some cases like expensive replications or very rare sample specimens, we have to concentrate more on the statistical or technical aspects since it will help to reduce the error.
2. Determining the Experiment Conditions
Identifying the potential factors in the experiment plays a vital role in every experimental design because their levels and combinations will be used as treatments for a disease. It is always advisable to get prior knowledge about the effects of the treatment or drug. If there is no information available, then a pilot study will help to identify the proper dosage. Ethical standard should be considered when dealing with living organisms because placebos are not always treated as controls. A control case is that it is the standard treatment that can be compared with the newer one. When there are more than two factors with different levels, then a factorial experiments can be used to identify the effectiveness of the drug. Following the factorial design for two genotypes (G and g) with 2 tissues (T, t) and replicated thrice (a, b, c).
Table 1
GTa | GTb | GTc |
Gta | Gtb | Gtc |
gTa | gTb | gTc |
gta | gtb | gtc |
In many designed experiments, there are situations with multiple factors with different levels, in that case seeking any statistical service will be helpful to minimise the cost of the experiment. Statisticians are always concerned with balanced designs. However, it is not always possible to get a balanced design in the biological experiments like genotype study and even in that case additional statistics expertise might help to conduct unbalanced experiments to reduce the expensive experiments.
Further, time is the important factor in the biological experiments. Often, the time referred in biological experiments is understood in different manner by the statistical experts. Suppose a biological sample, say a group of plants plucked at week 1 and 4, then this study will be considered as a longitudinal or repeated measurement design and statistician will assign a suitable analysis for this kind of data. By considering the time factor, one may reduce the false negative rates in the repeated measurement study. In addition, correlation is also an important part for any biological experiments, many of the longitudinal study often omit the correlation and this results in higher false positive rates. Thus, it is in the hand of biologists to correctly specify the importance of time in every designed experiment.
3. Determining the Platform of experiment
Budgets are the major cause for failure of many experiments. So, a proper platform should be figured out to conduct the research. Mostly research taken in lab will be expensive and one cannot attain a detailed information about the specimen. Thus, it is advisable to spend some quality time in deciding the best platform and best experiment for the study. In this situation, statistical service might be helpful in deciding the blocks of experiments either incomplete or complete block design with balanced or unbalanced cases.
5. Determining the Replicates
There are two types of replicates, one is biological replicates and the other is technical or statistical replicates. Biological replicates are used for arriving statistical inference whereas the technical replicates are the part of experiment objective and is a error in the process. It is advisable to get more biological replicates because higher the replicates lesser the errors. However, on certain cases, one cannot attain more replicates, as I said earlier; technical replicates will serve the purpose. Sample size is the key tool to reduce the error. This can be identified by using a power test under the null hypothesis. The following figure compares the effects of technical and biological replication. The black line in the graph shows the mean variation between the standard error and the biological (technical) variations and the red line is the effect of technical and biological variations respectively. The graphs shows that even though there exists a larger variation, the effect of biological (technical) replication coincides with the black line as the number of replicates increases. Thus, higher the replicates, lesser the error rates (Auer & Doerge, 2010).
Figure 1
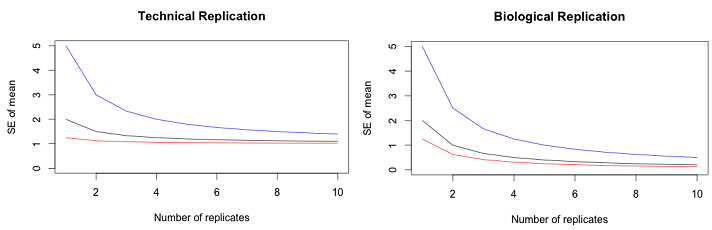
6. Determining the Material
It is often a common mistake carried out by many biological scientists to conduct the comparative experiments without proper genetic material. Suppose, if we are concerned about identifying the mRNA reading of two mice with equal age and weight. Then the result may not yield a valid mRNA. One can try cell sorter instead of using simply taking tissue Mrna (Nettleton, 2014).
7. Determining Measurement Design
Measurement design deals with how one should arrange the samples in the measurement equipment. It involves sample collection, sample correlation structure, block designs, etc.
In conclusion, a well-designed experiment should contain clear objectives and appropriate samples for the study and it should be reproducible. A good experimental design should minimise the cost and error and involves more replication. For conducting a valid experimental design, it is advisable to consult a statistical expertise or consult any data analysis service through online or offline mode to get better inference.
- Altman, N. S., & Hua, J. U. N. (2006). Extending the loop design for two-channel microarray experiments. Genetics Research, 88(3), 153–163. Retrieved from https://www.cambridge.org/core/journals/genetics-research/article/extending-the-loop-design-for-twochannel-microarray-experiments/1CED74BBC99B9488F92BC1E628C16A0E
- Auer, P. L., & Doerge, R. W. (2010). Statistical design and analysis of RNA sequencing data, pp. 405-416. Retrieved from https://www.genetics.org/content/185/2/NP.short
- Honaas, L. A., Altman, N. S., & Krzywinski, M. (2016). Study design for sequencing studies. In Statistical Genomics (pp. 39–66). Retrieved from https://link.springer.com/protocol/10.1007/978-1-4939-3578-9_3
- Kerr, M. K., & Churchill, G. A. (2001). Experimental design for gene expression microarrays. Biostatistics, 2(2), 183–201. Retrieved from https://academic.oup.com/biostatistics/article-abstract/2/2/183/278569
- Nettleton, D. (2014). Design of RNA sequencing experiments. In Statistical analysis of next generation sequencing data (pp. 93–113). Retrieved from https://link.springer.com/chapter/10.1007/978-3-319-07212-8_5