A review on basic statistical concepts of sampling and identifying study sample
Samples are the major part of any data analysis. As we all know samples represents the population. That is, for instance, collection of 100 samples represents the population say around 10,000. From the samples, one can identify the behaviour and make assumptions for the population. Well, that’s not the simple task. Because the sample size should be the ideal one while doing the statistical data analysis, it should not be too small or too large. In this blog, we will discuss about some of the basic concepts of sampling and what are the methods available to identify the sample.
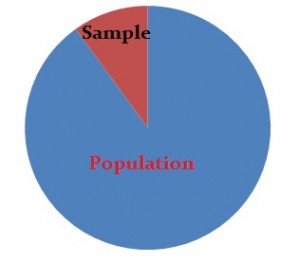
Let’s look into the most important concepts of sampling. They are
- Population or Universe – Population means the total number of items for which the information’sare desired whereas universe means the entire field of study. The population may be finite or infinite in nature. If it is finite, then there will be a countable number of elements and infinite means the observations or elements are countably infinite. Examples of finite population are number of students in a college, number of employees in a company, etc. The examples of infinite population include the stars in the sky, sand in a beach, etc.
- List of elements in a sample or the sampling frame – A sampling frame is a list of items from where the samples are to be taken. For conducting a survey on opinion, a frame can be a directory in a city. The most important thing is that you can adopt any sampling frame for sampling, but it should be a good representative of the population.
- Sampling plan or design – Sampling design is the important steps in getting a sample from the sampling frame. It gives the probability of the samples. Sampling data analysis plan includes defining the target population, suitable sampling techniques, identifying the sample size and executing the analysis.
- Statistic – A statistic measures the characteristic of the sample through the measure of central tendency and measure of dispersion, etc.
- Parameters – Parameter is what we estimate from the sample and is usually unknown. Parameters are identified using the statistic.
- Sampling Error – Accuracy of the statistical analysis often plays a vital role in any inference. Sometimes, rather often inaccuracy in the data may and those are otherwise called as sampling error. Usually, Sampling error = Frame error + chance error + response error. Statistician aims to reduce the error in the data.
- Precision – Precision and accuracy often treated as twins. Precision measures the reliability of the process. Precision can be identified using the confidence intervals or credible intervals.
- Level of Significance – Significance level is that the researcher fixes how much percentage of error they allowing for the analysis. It can 1%, 5% or even 10%. It is recommended to fix the level of significance as 5%.
How the samples can be collected?
In theory, there are two sampling procedure that are widely used. They are probability sampling and non-probability sampling. Probabilitysampling selects the sample in random manner whereas non-probability sampling uses non-random techniques. The following are the most common method of sampling that can be done with and without replacement.
- Simple random sampling – Selects the sample randomly with no specific order and the samples have equal probability.
- Cluster sampling – First selects the cluster sample in the form of groups or homogeneous clusters then a random sample is picked for the analysis. This type of sampling is used when the analyst doesn’t know about the observations or elements in a population instead some information about the subsets or groups are known.
- Systematic sampling – Selects the sample in a specific order like selecting every 10th observation from the data.
- Stratified sampling – Selects the sample as strata. That is, groups the data into homogeneous stratum and applying random or systematic sampling to get the samples from each stratum.
Apart from the above mentioned sampling methods, there are various types of sampling that are used in rare case. They are: adaptive sampling, bootstrap sampling, acceptance-rejection sampling, convenience sampling, sequential sampling, etc.
Now, we have understood some of the basic concepts of sampling and few methods of sampling techniques. Next, we will look into the sampling error. As I mentioned earlier, errors can happen at any stage of the analysis, either in the process of data collection, or data coding, or at the time of inference. When the data is collected like Census, then there would be no error in the outcome because calculating the error margin is quite impossible. When the data is of samples from the population then there will be a chance of errors. Lets understand this through an example. Suppose the analyst want to identify the percentage of people who like coffee in a auditorium with 1000 people, and the actual percentage is 19.3%, once analysing the data from the sample collected, the results came out as 19.36%. The margin of error is calculated as 1/√n which turns out to be a somewhere around 3% of margin error. In statistical practice, we used to say that when you have large sample, there will be less error. Thus, a proper designed experiment will reduce the error and give greater accuracy.
Often collection of large data analysing the same is difficult by the individual to study something about the population. For that nowadays, there are many statistical services or data analysis services available online to reduce the burden of the researcher. One can get a support from those statistical data analysis services from the data collection and further process.
In summary, a researcher should have a well designed plan before collecting the samples and have a clear idea about the sampling techniques. In addition, a researcher should know how to collect the samples and how well it will represent the population under study. Hope this blog will help the researcher to understand the sampling process and some of the common types of sampling techniques and how and when to use the same.
References:
- Raghunath Arnab (2017). Survey Sampling Theory and Applications. Academic Press.
- Sharon L. Lohr (2019). Sampling: Design and Analysis: Design and Analysis (Second Edition). Brooks/Cole.
- Sebastian Baltes and Paul Ralph. (2020). Sampling in Software Engineering Research: A Critical Review and Guidelines. ACM Trans. Softw. Eng. Methodol.
- Inez M. Zwetsloot & William H. Woodall (2019): A review of some sampling and aggregation strategies for basic statistical process monitoring, Journal of Quality Technology.