Model-based clustering using a Bayesian approach for binary panel Probit models with examples
Model-based clustering using Bayesian approach for binary panel Probit models
In common Statistical Analysis, the classical estimation for various situations may be invalid, in the sense that it may be lead to misinterpretations. To deliver more appropriate results for the study, Bayesian paradigms have emerged. In Bayesian paradigm, each explanatory variable are assumed to be a random variables. It involves formulating a suitable prior distribution for the data under study and result will yield in a posterior distribution. Nowadays, with the advent of computational practice, Bayesian modelling becomes a interesting area of research in all the field of science. Thus, in this blog, I will discuss about a Bayesian approach for Probit Models through clustering approach as discussed in Aßmann and Hogrefe (2011).
What is a probit model?
A probit model is a type of Regression Model in which the response variable can take only binary outcomes (eg. yes or no, married or unmarried, male or female, etc.,). Generally, Probit model are considered as class of Generalized Linear Mixed Models especially in the panel study. Let us now, discuss the issues affecting the probit model and how to select a better model using Bayesian perspective.
Endogeneity and Heterogeneity in the probit or logit models are an important issue in estimating the variables since it estimates the cumulative functions. However, heterogeneity especially in non-linear model yields an attention to the researchers in recent years to provide better estimates to the variables. And, Bayesian flavour has been increasing in this area to provide appropriate results. Clustering approach is served as a supporting tool for modelling the latent heterogeneity in the probit models. However, the question of choosing the number of clusters still persists. An approach of using marginal likelihood and a cross-validation technique is adopted to identify the number of clusters and a simulation study is adopted to assess these approaches in Aßmann and Hogrefe (2011). Literature is abundant for estimating the latent heterogeneity through clustering approach using fixed and random effects (See, Cameron and Trivedi (2005), Lancaster (2000)). The purpose of using Bayesian estimation is that it can be used to estimate even when the sample size is small and it has an ability to identify the unknown or uncertain parameters correctly through a Posterior Distribution.
Now lets discuss the key points of the techniques adopted in this paper. Firstly, the priors are chosen with normal and dirichlet distribution for the parameters under study and are presented in the following table. The non-random and random cluster specification priors are used for the simulation and the last part is used for the empirical study.
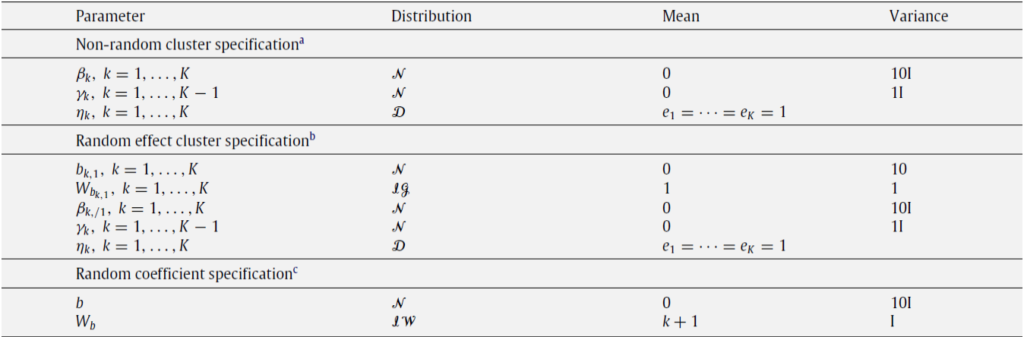
Estimation procedures adopted for the simulation study are Gibbs sampling, MCMC sampling, and Bridge sampling using maximum likelihood and Cross-Validation technique and conducted eleven scenarios for this purpose. A comparison of the log-likelihood of the marginals and the out-of-sample prediction is presented with cluster and stratified clustering technique and the results are discussed in the references therein.
The proposed stratified clustering Bayesian probit model is illustrated with a real time dataset from Bertschek and Lechner (1998). The data involves investments of 1270 competitive firms from the years 1984 to 1988. The empirical study indicated that the proposed method provides better model specifications. Two cluster specific random effects (RE) specifications with uncorrelated and correlated random effects with stratified and unconditional probabilities are considered to model the latent heterogeneity using binary probit model using Bayesian estimation and the comparison results of the models are depicted in the following table.
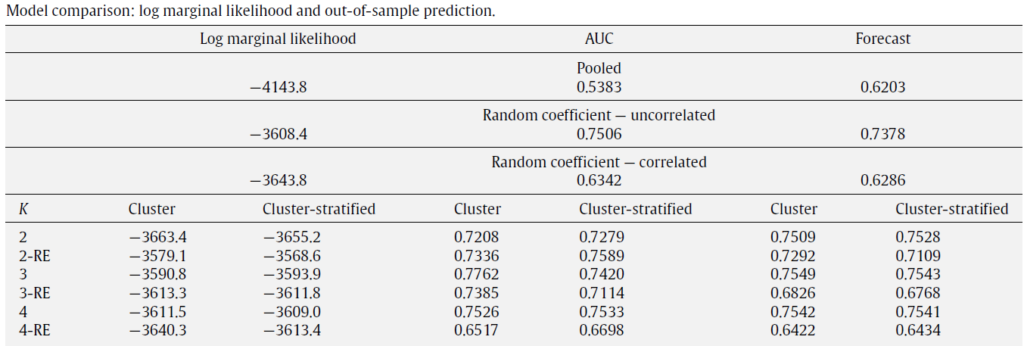
From the above table, it is clear that random coefficient specification is preferred as normally described in Jefferys’ scale. Also, the uncorrelated random effects shows a better characterization of latent heterogeneity.
Next part is to identify the number of clusters, for that the author used stratified and non-stratified probabilities and it yields similar results in estimating via marginal likelihood, whereas in the out-of-sample method AUC measure indicated the better cluster strategy to use i.e the non-stratified clusters and the Bayesian estimation for the preferred specifications are tabulated and it concludes that the firm has no positive effect. The variables substantiate the effect of the firm innovation is that the log scale, investment and the firm size among the other variables.
In conclusion, the issue of model selection under the latent heterogeneity is analyzed using the Bayesian probit model via clustering approach and the results of the study revealed that the model selection using marginal likelihood is preferred in Bayesian point of view. Also, the proposed stratified clustering technique yields better performance in different scenarios than compared with classical non-stratified clustering method. The illustrative example revealed that the there exists a strong evidence in capturing the latent clusters using this latent heterogeneity methods. However, the author conclude that in out-of-sample case, the stratified clustering doesn’t give satisfactory results because of the AUC measure and concludes that there is a need for more appropriate methodology to give consistent results in model selection process.
see also here
- Aßmann, C., 2007. Determinants and costs of current account reversals under heterogeneity and serial correlation. Economic Working Paper No. 2007-17, CAU Kiel.
- Aßmann, C., Hogrefe, JS., 2011. A Bayesian approach to model-based clustering for binary panel probit models. Computational Statistics and Data Analysis, 55 261-279.
- Bertschek, I., Lechner, M., 1998. Convenient estimators for the panel probit model. Journal of Econometrics 87 (2), 329–372.
- Cameron, C., Trivedi, P., 2005. Microeconometrics: Methods and Applications. Cambridge University Press.
- Chakraborty, S., 2009. Bayesian binary kernel probit model for microarray based cancer classification and gene selection. Computational Statistics & Data Analysis 53, 4198–4209.
- Chen, J., Khalili, A., 2008. Order selection in finite mixture models with a nonsmooth penalty. Journal of the American Statistical Association 103 (484), 1674–1683.
- Congdon, P., 2006. Bayesian model choice based on monte carlo estimates of posterior model probabilities. Computational Statistics & Data Analysis 50, 346–357.
- Dunson, D., Herring, A., Siega-Riz, A., 2008. Bayesian inference on changes in response densities over predictor clusters. Journal of the American Statistical Association 103 (484), 1508–1517.
- Fraley, C., Raftery, A., 2002. Model-based clustering, discriminant analysis, and density estimation. Journal of the American Statistical Association 97, 611–631.
- Gamerman, D., Lopes, H., 2006. Markov Chain Monte Carlo: Stochastic Simulation for Bayesian Inference. Chapman & Hall/CRC Press.
- Geisser, S., Eddy, W., 1979. A predictive approach to model selection. Journal of the American Statistical Association 74 (365), 153–160.
- Yao, W., Lindsay, B., 2009. Bayesian mixture labeling by highest posterior density. Journal of the American Statistical Association 104 (486), 758–767.